Artificial intelligence (AI) is rapidly reshaping the business landscape, and at the forefront of this transformation is Generative AI (GenAI), an exciting frontier within the AI domain. Fuelled by the immense power of large language models (LLMs) trained on vast datasets, GenAI can comprehend natural language prompts and generate high-quality content. This technology can potentially revolutionize business operations, positioning AI models as central drivers of enterprise success. As per Gartner’s predictions for 2025, 30% of enterprises will have integrated AI-augmented development and testing strategies, a substantial leap from the 5% recorded in 2021.
Generative AI, a subset of AI and deep learning, is focused on creating novel content and ideas across various domains, encompassing text, images, audio, and video. The widespread adoption and potential value of Generative AI are accelerating its application in experimental, consumer, and, increasingly, enterprise use cases. While much attention has been directed towards consumer applications, business opportunities are vast, and some are already being realized. However, questions persist regarding how individuals and enterprises can harness Generative AI to achieve efficiency gains, enhance products, create novel experiences, or drive operational transformation. Likewise, the commercialization of Generative AI and sustainable business model development are still evolving. To begin, let’s comprehend the fundamental characteristics and essential components that constitute Generative AI.
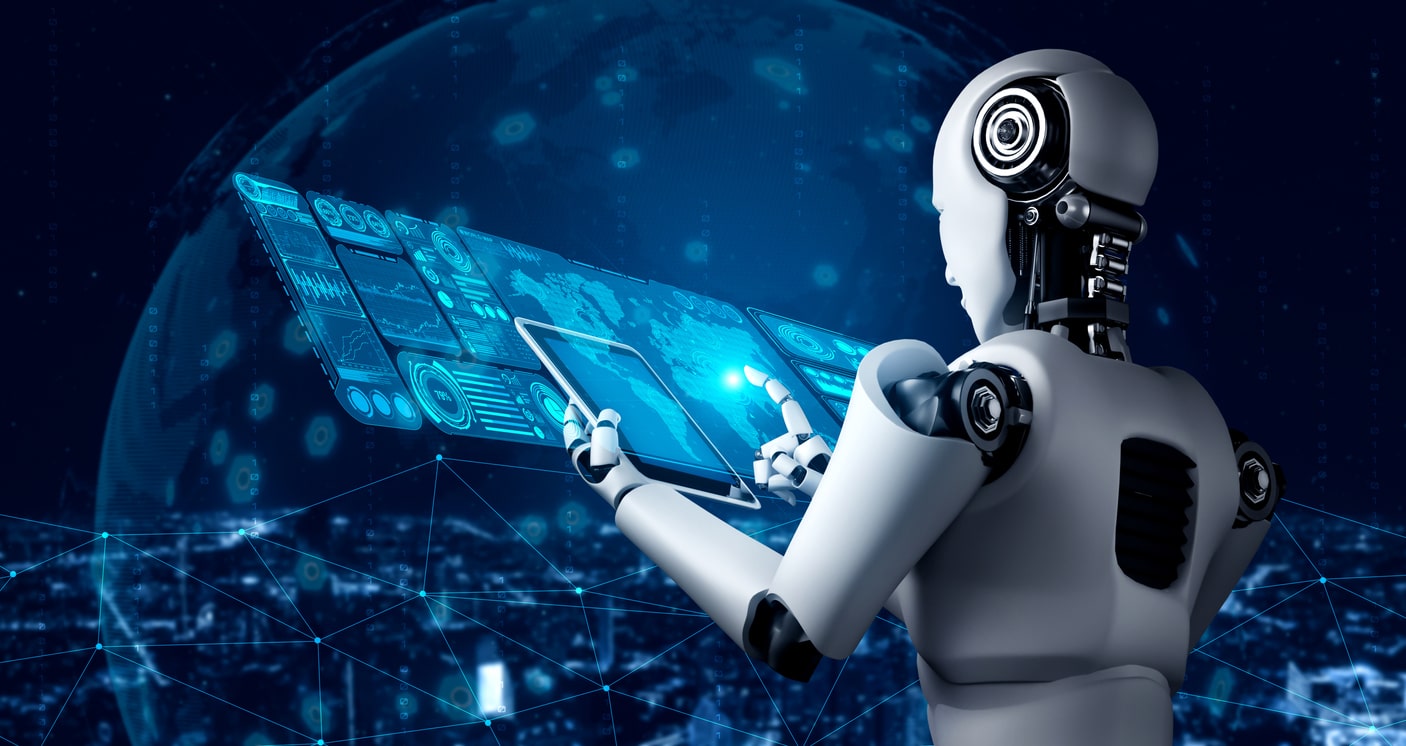
Here’s What You Will Learn in This Blog
- Key Characteristics and Components of Generative AI
- How to Improve Employee Productivity
- AI / Gen AI: Use-Cases
- How to Streamline Business Operations
- How to Enrich Customer Experience
- How to Speed up Creativity and Innovations
- Learn About Lowest Complexity Scenarios
- How to fine tune Existing LLMs for Mid-Level Complexity
- Build your own Models and Support Multiple Models
- Frequently Asked Questions (FAQs)
Key Characteristics and Components of Generative AI

Creation of New Content and Ideas
Generative AI systems can generate original and creative content distinct from direct copies of existing data. This includes generating coherent text, lifelike images, musical compositions, and engaging natural language conversations.

Subset of Deep Learning
Generative AI is firmly rooted in deep learning techniques, a specialized branch of machine learning. Deep learning models, particularly Artificial Neural Networks (ANNs), are pivotal in powering Generative AI systems.

Reliance on Large Pretrained Models
Generative AI models depend on extensive pre-trained models, often called ‘foundation models’ (FMs). These models have undergone training on comprehensive datasets from the internet, accumulating knowledge and patterns from diverse sources. These FMs serve as the foundation upon which new content generation is built, underpinning the versatility and creativity of Generative AI.
The convergence of AI and Generative AI is reshaping how businesses approach innovation, content creation, and customer engagement. As GenAI continues to evolve and mature, it promises to be a driving force behind the next wave of enterprise transformation.
Artificial Intelligence, including Generative AI (GenAI), promises to revolutionize internal operations, leading to substantial cost savings and enhanced efficiency. Here are some use cases of how to use artificial intelligence in business to achieve objectives:
Improve Employee Productivity
Harnessing the potential of artificial intelligence in business, both AI and GenAI emerge as transformative forces in significantly boosting employee productivity. Companies can achieve this by automating routine tasks and offering intelligent support. In the realm of improved employee onboarding, businesses can exemplify this with real-world instances. For instance, they can streamline the onboarding process by leveraging AI-driven chatbots that provide new employees instant answers to their queries, ensuring a smoother transition into the organization.
Conversation-Style Search of Intranet Content:
Implement a conversational search interface within your organization’s intranet, allowing employees to find information quickly and efficiently. This conversational approach plays a vital role in artificial intelligence in business, enabling users to ask questions in natural language, receive relevant results, and improve knowledge accessibility.
Content Summarization:
AI-powered content summarization tools can distill lengthy documents, reports, or articles into concise summaries, saving time for employees who need to extract critical information swiftly.
Productivity, Including Text to Speech:
Utilize AI-driven productivity tools that offer features like text-to-speech conversion. Employees can have documents read aloud to them, enabling multitasking, and improving accessibility for visually impaired individuals. Using artificial intelligence in business, companies can unlock substantial cost savings and significantly boost operational efficiency. These technologies empower employees to work smarter and focus on tasks that require their expertise, ultimately driving the organization toward higher productivity and success.
AI / Gen AI: Use-Cases
Cost Saving and Internal Efficiency
Revenue Growth and User Experience
AI plays a pivotal role in optimizing day-to-day business processes. It can automate repetitive tasks, such as data entry and report generation, reducing human error and improving operational efficiency. AI-powered chatbots can handle customer inquiries and employee support and even assist in managing internal workflows. Let’s now delve into the application of artificial intelligence in business operations:
Streamline Business Operations
1. Insights from Structured/Unstructured Data:
AI excels in data analysis, providing valuable insights from structured and unstructured data sources. Machine learning algorithms can uncover patterns, trends, and anomalies within data, enabling data-driven decision-making. This is particularly useful in market analysis, customer sentiment analysis, and risk assessment.
2. Supply Chain Optimization:
AI-driven supply chain optimization involves predictive analytics to forecast demand accurately, manage inventory efficiently, and optimize logistics routes. This results in reduced costs minimized stockouts, and improved delivery times. AI can also identify potential disruptions and recommend proactive measures.
3. Business Function Enhancements – Sales/Operations/Executives:
AI augments various business functions. In sales, AI-powered predictive analytics can identify potential leads and personalize sales strategies. For operations, AI monitors equipment health in real time, predicting maintenance needs to prevent downtime. At the executive level, AI-driven dashboards provide comprehensive insights, aiding strategic decision-making.
4. AI-Supported Maintenance Use-Cases:
AI enhances predictive maintenance by analyzing equipment data and identifying signs of potential failures. This proactive approach minimizes downtime, reduces maintenance costs, and extends the lifespan of assets. It is commonly used in manufacturing, energy, and transportation sectors.
5. Industry 4.0 Use-Cases
Industry 4.0 leverages AI and IoT (Internet of Things) to create smart factories. AI monitors equipment adjusts production lines in real time and optimizes resource allocation. It leads to increased efficiency, reduced waste, and improved quality control. Some artificial intelligence in business examples include AI-driven smart buildings that can enhance energy efficiency, security, and occupant comfort. AI-controlled HVAC systems adjust temperatures based on occupancy, saving energy. Facial recognition and sensor-based security systems improve safety. AI schedules meeting rooms in smart offices and optimizes lighting and heating based on employee preferences.
AI’s adaptability and capability to analyze data and make predictions make it a valuable tool for streamlining various aspects of business operations. Whether it’s automating tasks, optimizing supply chains, enhancing decision-making, or improving maintenance, Business AI has a broad range of applications that can drive efficiency and cost savings in diverse industries.
GenAI can be used to personalize customer interactions, create engaging content, and develop new products and services. Let’s now explore how AI can significantly contribute to revenue growth and enhance user experiences, particularly in terms of enriching customer interactions:
Enrich Customer Experience
AI plays a pivotal role in elevating customer experiences by providing personalized, efficient, and responsive interactions. Here’s how:
1. Chatbots / Conversational Interfaces
AI-driven customer experience enhancements begin with understanding customer behavior and preferences. AI algorithms analyze historical data to anticipate customer needs and deliver tailored experiences. Chatbots powered by AI are available around the clock to engage with customers in natural language. They can quickly respond to inquiries, guide users through product selection, troubleshoot issues, and even assist in completing transactions. These chatbots offer a seamless and efficient way to engage with customers.
2. Virtual Assistants
Virtual assistants are AI-driven interfaces that go beyond basic chatbots. They can provide comprehensive support and perform tasks such as scheduling appointments, ordering products, and providing in-depth information. Virtual assistants are designed to mimic human-like conversations, offering a more engaging and personalized experience.
3. AI Enhanced Customer Support
AI enhances customer support by automating routine inquiries and issue resolution. It can respond immediately to frequently asked questions, create support tickets, and escalate complex issues to human agents when necessary. This streamlines support processes, reduces response times, and ensures consistent service quality.
4. Omni-channel Interactions
AI enables seamless omnichannel experiences, where customers can interact with businesses through channels, including websites, mobile apps, social media, and messaging platforms. AI ensures the customer’s journey is consistent and coherent across these channels, creating a unified and positive experience.
Speed up Creativity and Innovations
AI’s contribution to creativity and innovation is increasingly significant. It empowers individuals and organizations to ideate, create, and innovate in ways that were not unimaginable before. Here’s how:
Image/Voice/Video Related Use-Cases:
• Content Generation: AI can automatically generate content like articles, reports, and creative fiction. This streamlines content creation, making it more efficient and scalable.
• Image Manipulation: AI-driven image processing tools can enhance and manipulate images to create stunning visuals. This includes retouching photos, generating art, and creating realistic images from scratch.
• Voice Synthesis: AI-based text-to-speech technologies enable the creation of realistic, human-like voices. This is invaluable for generating voiceovers, audiobooks, and accessibility features.
• Video Editing: AI-driven video editing tools can automate editing, making it faster and more accessible for creators. They can even assist in generating video content from text descriptions.
AI can significantly aid in writing marketing content, ensuring it’s engaging, informative, and tailored to the target audience:
• Content Personalization: AI analyzes user behavior and preferences to deliver personalized marketing content, improving engagement and conversion rates.
AI-Enhanced Media Services:
• Image Creation: AI can generate images, illustrations, and designs tailored to specific needs. For instance, it can create custom graphics for marketing campaigns or social media posts.
• Music Creation: AI can compose music, generate soundtracks, and even remix existing tracks to fit various contexts, from video production to advertising.
• Video Production: AI can assist in creating videos by automatically generating animations, adding special effects, or even providing video editing recommendations.
AI’s role in accelerating creativity and innovation is transformative. It offers various tools and capabilities, enabling individuals and businesses to create, experiment, and innovate faster and more efficiently. Whether it’s generating content, manipulating media, or personalizing marketing strategies, AI empowers creativity and fosters innovation across diverse industries and creative domains.
Let’s explore the adoption patterns of Generative AI, beginning with those that pertain to the lowest complexity scenarios.
Lowest Complexity Scenarios
• Prompt Engineering: This approach involves crafting specific and well-structured prompts to guide Gen AI in generating desired content. By refining prompts, users can achieve more predictable and controlled outcomes.
• Retrieval Augmented Generation (RAG): RAG combines retrieval models with generative models, improving the quality and relevance of content generation. It allows users to provide better prompts and retrieve relevant information from large datasets.
• Integrate with Existing Systems: Gen AI can be seamlessly integrated with existing enterprise systems and workflows. This integration ensures that Gen AI becomes integral to the organization’s daily operations. This approach caters to a broad range of enterprises and their Gen AI use-cases, offering adaptability and versatility in addressing various business needs.
Fine-Tuning Existing LLMs for Mid-Level Complexity
Fine-tuning existing Large Language Models (LLMs) is a crucial technique in Natural Language Processing (NLP) when dealing with mid-level complexity tasks. This approach is precious when there is substantial data to work with. Let’s delve into the details of fine-tuning LLMs for mid-level complexity tasks.
Techniques for Fine-Tuning:
1. Data Augmentation: Fine-tuning LLMs often involves augmenting the training data with additional examples. This process helps the model generalize and improve its performance on specific tasks.
2. Task-Specific Data: In mid-level complexity tasks, having task-specific data is essential. Fine-tuning entails providing the model with labeled examples relevant to the task at hand, allowing it to adapt to the nuances of the problem.
3. Hyperparameter Tuning: Adjusting hyperparameters such as learning rates, batch sizes, and optimization algorithms is part of fine-tuning. These adjustments help optimize the model’s performance for a specific task.
4. Layer Adaptation: Some fine-tuning approaches involve modifying specific layers of the LLM. For instance, adjust the output layer to match the number of classes in your task.
5. Transfer Learning: Fine-tuning often begins with a pre-trained LLM, leveraging its knowledge of general language understanding. This approach saves time and computational resources.
When is fine-tuning needed?
Fine-tuning becomes necessary when dealing with mid-level complexity tasks, where a substantial amount of task-specific data is available, and the model’s pre-trained knowledge can be adapted to the task’s requirements. These tasks include sentiment analysis, text classification, language translation, or summarization.
• Improved Task Performance: Fine-tuning allows LLMs to excel in specific tasks by adapting their language understanding capabilities.
• Reduced Training Time: Fine-tuning is typically faster than training a model from scratch since the pre-trained model already has a strong foundation in language understanding.
• Cost-Effective: Businesses can save on computational resources and time by leveraging pre-trained models and optimizing them for specific tasks.
Basically, fine-tuning existing LLMs is a powerful technique for mid-level complexity NLP tasks. Organizations can adapt pre-trained models to suit their unique needs by providing task-specific data and carefully adjusting hyperparameters, resulting in improved performance and cost-effective solutions.
As we delve deeper into the adoption patterns of Generative AI, we arrive at high complexity, where the enterprises find their unique challenges. Let’s now explore the dynamics of this strategy in more detail.
Build your own Models and Support Multiple Models
• Most Applicable to Largest Enterprises: Building proprietary models and offering robust model support is especially pertinent for the largest enterprises. These organizations operate on a bigger scale and often contend with exceptionally intricate language-based tasks. Whether managing vast customer interactions, scrutinizing complex legal documents, or navigating complicated technical content, proprietary models and dedicated model support offer highly specialized solutions to these colossal challenges.
• Creating Proprietary Models: Large enterprises often possess the resources and in-house expertise to construct their proprietary NLP models from the ground up. This approach empowers them to design models that are precisely tailored to their unique requirements, including specialized industry terminologies, domain-specific nuances, and proprietary data structures.
• Comprehensive Model Support: In the complex operational landscape of large enterprises, the need for versatile model support is paramount. These organizations often require comprehensive support for many models, each intended for specific tasks or domains. This support encompasses model training, maintenance, monitoring, and continuous improvement to ensure peak performance across the model portfolio.
• Benefits for Large Enterprises:
Customized Solutions: Building proprietary models allows large enterprises complete control over model design and fine-tuning, ensuring alignment with industry-specific terminologies and contextual nuances. This level of customization enhances model accuracy and relevance.
• Versatility and Scalability: Large enterprises thrive on versatility and scalability. The ability to build and support proprietary models means they can create a diversified model ecosystem that addresses the multifaceted challenges of their operations, ensuring adaptability and efficiency.
• Exceptional Performance: High-complexity tasks demand exceptional performance. Proprietary models, built to exact specifications, can deliver the precision and accuracy required for critical business operations, informed decision-making, and regulatory compliance.
• Data Control and Security: Data control and security are paramount concerns in large enterprises. Building proprietary models allows them to maintain complete control over their data while leveraging the benefits of AI and NLP technologies.
Building proprietary models and offering robust model support is a strategic imperative for the most prominent enterprises grappling with high-complexity NLP challenges. This approach empowers large enterprises to maintain their competitive edge and drive innovation within their respective industries by enabling deep customization, scalability, and stringent data control.
Now, let’s take you through the implementation plan involving the end-to-end framework for harnessing GenAI in the enterprise:
Customers can realize many advantages through the Nsight solution, a cutting-edge platform designed to empower businesses and steer them toward transformative outcomes. The capabilities offered by Nsight unlock a world of opportunities for customers, and here’s a comprehensive look at how they can benefit:
End-to-End Framework (Cloud Platform, Application):
Streamlined Integration: Nsight offers an end-to-end framework that seamlessly integrates with existing systems and infrastructure, ensuring a smooth transition to AI-driven solutions.
• Comprehensive Solutions: Our solution spans both the cloud platform and application layers, providing a holistic approach to AI implementation that covers all your operational needs.
Proof of Concept (POC):
• Customized Testing: With Nsight’s Proof of Concept (POC) phase, customers can test AI solutions tailored to their unique requirements. This ensures that the proposed AI strategies align perfectly with their business goals.
• Risk Mitigation: POCs allow customers to evaluate the feasibility and effectiveness of AI initiatives before committing to full-scale deployment, minimizing risks associated with adoption.
Solution Ready for Commercial Scale-Up:
• Seamless Transition: Nsight ensures a smooth transition from POC to commercial deployment. Once the POC phase is successfully completed, the solution is ready for rapid scale-up within the customer’s organization.
• Time and Cost Efficiency: Commercial scale-up is streamlined, saving customers valuable time and resources as they move from testing to full implementation.
Scalability and Implementation Support:
• Scalability: It can effortlessly scale to accommodate increased data volumes, user demands, and evolving business needs.
• Dedicated Support: Our team provides ongoing implementation support to ensure that Nsight continues to deliver value as your organization expands and evolves.
Enterprise-Wide AI Solution:
• Holistic AI Integration: Nsight serves as an enterprise-wide AI solution, capable of addressing diverse use cases across various departments, from supply chain management to customer relations and beyond.
• Consistency and Integration: Nsight ensures consistency in AI implementation throughout the organization, promoting a unified and integrated approach to leveraging AI technologies.
Nsight goes beyond being a mere AI solution – it’s a strategic asset that empowers businesses to harness the full potential of AI. From end-to-end integration and POC testing to seamless scale-up and enterprise-wide deployment, Nsight is dedicated to driving innovation, efficiency, and competitive advantage for our valued customers.
Schedule a Consultation and Experience the Future of AI-Driven Success with Nsight!
Frequently Asked Questions (FAQs)
Artificial Intelligence in Business refers to applying AI technologies to enhance and streamline various business processes, leading to improved efficiency and decision-making.
Businesses can leverage AI to automate tasks, gain insights from data, enhance customer experiences, and optimize operations.
Examples include chatbots for customer support, predictive analytics for sales forecasting, recommendation systems for personalized marketing, and AI-driven data analysis for insights.
AI helps in decision-making by providing data-driven insights, automating repetitive tasks, and offering predictive analysis to inform strategic choices.
Advantages include improved efficiency, cost savings, enhanced customer experiences, better decision-making, and competitive advantage.
Yes, challenges include data privacy concerns, integration with existing systems, and the need for specialized AI talent.
AI is critical in optimizing business processes by automating routine tasks, reducing errors, and enabling data-driven improvements.
Benefits include increased productivity, faster decision-making, better customer insights, and the ability to stay competitive in a rapidly changing market.
Disadvantages may include high initial costs, potential job displacement, and ethical concerns surrounding AI applications.
About the Author
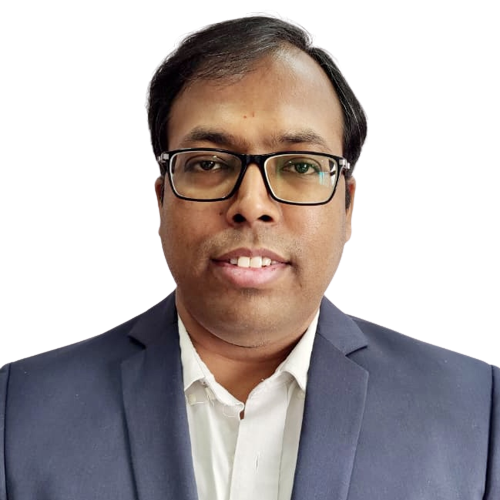
Deepak Agarwal, a digital and AI transformation expert with over 16 years of experience, is dedicated to assisting clients from various industries in realizing their business goals through digital innovation. He has a deep understanding of the unique challenges and opportunities, and he is passionate about using cutting-edge technologies to solve real-world business problems. He has a proven track record of success in helping clients improve operations, increase efficiency, and reduce costs through emerging technologies.